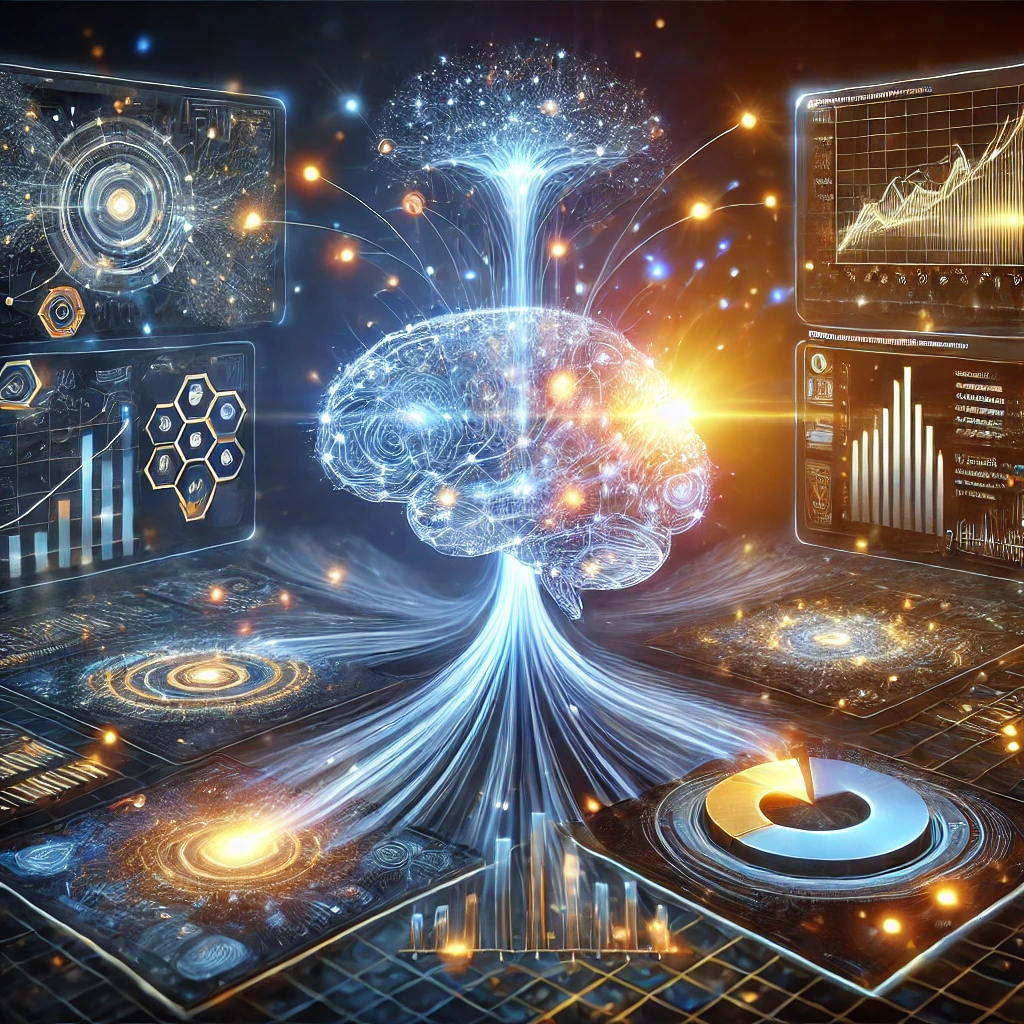
At the current pace of this digital landscape, harnessing real-time data has become a game-changer for businesses. As the CEO of Meroxa, I've witnessed firsthand how generative AI not only enhances data processing but fundamentally reshapes how organizations extract value from their continuous streams of information. Whether you're a mid-market enterprise or a Fortune 1000 company, embracing these technological advancements leads to transformative improvements in decision-making and operational efficiency. In this post, I'll explore how integrating large language models (LLMs) into data pipelines and leveraging conversational analytics are setting new standards for real-time applications.
Integrating LLMs into Real-Time Data Workflows
The New Era of Data Pipelines
At its core, integrating LLMs into data workflows embeds intelligence throughout the data processing lifecycle—from ingestion to analysis. Traditional data pipelines focused on collecting and transforming data for batch processing. Now, with generative AI, organizations are shifting toward models that transform real-time data into actionable insights instantly.
Consider a financial institution processing millions of transactions per minute. By incorporating a GPT-based LLM into its pipeline, the institution can automatically flag unusual patterns, assess risks in real time, and generate concise summaries of emerging market trends. This capability enhances operational agility while empowering decision-makers with immediate insights into potential risks and opportunities.
Real-World Example and Benefits
In retail—where consumer behavior and market sentiment shift rapidly—companies can integrate generative AI into streaming data feeds to monitor social media trends and point-of-sale transactions simultaneously. The LLM analyzes vast data volumes, creating real-time summaries that highlight changes in consumer preferences and emerging product trends. This enables marketing teams to quickly adjust campaigns while supply chain managers optimize inventory based on immediate demand signals.
Key Benefits:
- Speed and Agility: Real-time insights enable instant responses to emerging events.
- Resource Optimization: Automated summarization frees skilled analysts to focus on strategic work.
- Scalability: Modern AI models efficiently handle high-volume streaming data.
- Improved Accuracy: Continuous model updates ensure insights stay timely and relevant.
Technical Workflow Diagram
Let me show you how a modern real-time data pipeline integrates LLMs using Meroxa for data ingestion and processing - this diagram breaks down the key components and how they work together:
Raw data flows from multiple sources through Meroxa's platform for ingestion and preprocessing, then through LLM analysis, finally generating automated insights for dashboards and alerts.
Challenges to Consider
While the benefits are significant, integrating LLMs into real-time pipelines comes with key challenges. Data quality is paramount—the system requires clean, consistent, and secure information to function effectively. Processing real-time data through large models demands substantial computational power. To address this, organizations need scalable infrastructure and may need to implement edge computing to reduce latency. Additionally, robust security and data governance protocols must protect sensitive information throughout its journey.
The Rise of Conversational Analytics
From Dashboards to Dialogues
The business intelligence (BI) landscape is evolving. Traditional dashboards and static reports are giving way to conversational analytics platforms that let users interact with data through natural language queries. Instead of waiting for detailed reports, executives can now ask questions like "What were our top-selling products last month, and what factors drove their success?"—and receive immediate, context-rich responses powered by GPT-based foundation models.
Enhancing User Experience and Decision-Making
Conversational analytics democratizes data access across organizations. Advanced data analysis is no longer confined to technical teams—executives, managers, and frontline employees can now engage with data using everyday language. This accessibility speeds up decision-making by delivering insights promptly in a user-friendly format.
Interactive, chat-like interfaces transform data interaction into a dynamic dialogue. This approach cultivates data literacy throughout the organization, preventing insights from being siloed within select groups. By removing technical barriers, businesses enable their entire workforce to participate in data-driven decision-making.
Technical Architecture for Conversational Analytics
In this architecture, a natural language query initiated by a user is processed by a conversational interface that leverages a GPT-based model. The query is then further refined and processed before data is retrieved and transformed by Meroxa’s real-time data store. This transformed data is used to generate an immediate, actionable response for the user.
Driving Business Value
For technical business decision-makers, the value proposition of conversational analytics is clear and compelling:
- Enhanced Accessibility: Natural language queries eliminate dependence on technical specialists, democratizing data insights across the organization.
- Faster Insights: Real-time, interactive querying bridges the gap between data generation and action—essential in today's fast-moving markets.
- Cost Efficiency: Automated analysis reduces the operational costs traditionally associated with BI systems.
- Competitive Edge: Organizations that quickly interpret and act on real-time data gain a decisive market advantage.
Strategic Implications for Business Leaders
Embracing the Future Today
The integration of generative AI into real-time applications isn't just a technological trend—it's a strategic imperative. For technical business decision-makers, the ability to extract immediate, actionable insights from data streams drives revenue growth, enhances operational efficiency, and mitigates risks.
At Meroxa, we empower organizations to seamlessly integrate these cutting-edge technologies into their existing data workflows. By connecting real-time data ingestion with AI-driven analytics, we help businesses unlock generative AI's full potential.
Overcoming Barriers and Building a Data-Driven Culture
While adopting generative AI presents challenges—from data quality to computational demands—the rewards far outweigh the investment. Enhanced decision-making, operational agility, and market competitiveness await organizations that commit to this transformation. Success hinges on fostering a culture that embraces data-driven insights and invests in the right infrastructure and talent.
Whether you're just beginning your digital transformation or looking to accelerate it, now is the time to explore how generative AI can revolutionize your data strategy. The strategic advantages of streamlined data pipelines and intuitive analytics tools are undeniable.
Conclusion
In today's world of constant change and endless data flows, generative AI in real-time applications isn't optional—it's essential. By combining LLMs with data pipelines and conversational analytics, businesses can achieve unprecedented levels of insight, efficiency, and agility. At Meroxa, we envision data not just as something to collect, but as a strategic asset that powers informed decisions and creates lasting competitive advantages.
I urge technical business decision-makers, from mid-market enterprises to Fortune 1000 companies, to embrace these transformative technologies. This step will position your organization to not just succeed in a data-driven world, but to pioneer the next wave of business innovation.
Follow us on Twitter, LinkedIn, and YouTube for more insights and updates!